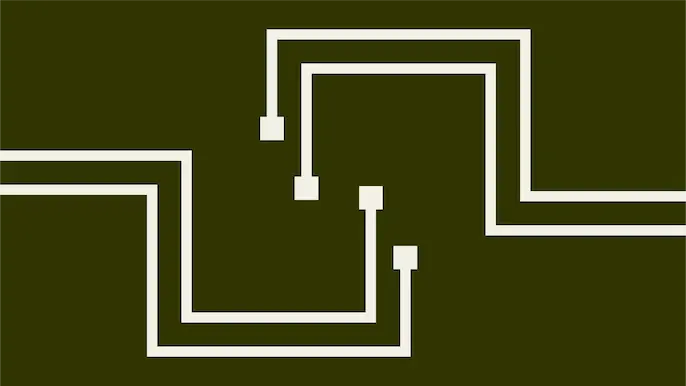
Navigating Cybersecurity Innovation and Maturity in 2021
The word innovate, as defined by Merriam-Webster, means “to make changes or to do something in a new way.” In cybersecurity, this definition goes much deeper. The goal of many, if not all, cybersecurity organizations today is to be innovative in its space, or at least be more innovative than its adversaries. That raises the question, how do we define and drive cybersecurity innovation today?
When building a cybersecurity organization that aims to disrupt the industry, there is much to consider. Drawing from my experience working with many early-stage cybersecurity and risk management software companies, I sat down with NetSPI managing director Nabil Hannan on the Agent of Influence podcast to explore how to define cybersecurity innovation, evaluate risk factors, achieve program maturity, and more. In this blog post, I will highlight and expand on key insights from the discussion. You can listen to the full podcast episode here.
Innovation in commercial software for enterprises originates from companies that are not market leaders.
I’ve always believed that innovation in cybersecurity originates with early-stage companies that are not market leaders. Sure, market leading software companies have tremendously talented and capable people. However, innovation requires making mistakes and adjustments based on lessons learned. Market leaders have to allocate development and product management resources to meet the needs of the broadest part of the market, and prioritize the needs of their shareholders, investors, and customers. If they do this, they are successful and able to sustain market leadership. Market growth is dependent on the ability to sell software to the largest part of the market (the most customers). More customers means higher market leadership and, in turn, happy investors, shareholders and employees.
The same economic rules apply to the enterprise market for cybersecurity products. The difference is that the broadest or largest part of the enterprise cybersecurity market is the least sophisticated in practices and controls. Therefore, innovation is not necessary for success as many enterprises often make buying decisions based on analysis of market leaders and what other respected enterprises decide to do. Early-stage cybersecurity companies that develop game changing capabilities do so because they can afford to take risks that could result in failure but also could result in innovation – market leaders can’t afford to take the same risks. The early-stage companies that have success with developing truly game changing capabilities for an enterprise to survive and thrive by creating friction for threat actors. Several large enterprises encourage early adoption of innovative capabilities for cybersecurity to keep up with the evolution of threat actor tactics and develop breakthrough technologies for enterprise protection and resilience. Their culture allows them to take risks to better manage risks for the enterprise.
Innovation is sustained failure.
For any cybersecurity function, it is important to have a culture that supports innovation in control design. The way I define it is, “innovation requires fast failure.” Before you challenge that assumption, let me explain that innovation comes from adjustments in assumptions that are made as a result of obstacles that are discovered, causing pivots. Pivots are changes in direction from applying the sometimes-painful lessons learned after a failure.
Innovation is a constant iteration of small failures. The failures have to be acknowledged, understood, and then the lessons learned have to be applied – that’s the pivoting part. [Bonus: if you can learn from somebody else’s failure, even better.] This is the normal cycle for innovation. I’m certain that any innovation in consumer digital technology over the last 30 years is a direct result of some level of failure.
The learning process, specifically in control design, is in a constant evolution and is always changing. On the enterprise security side, we have to remember that threat actors always change their tactics. It’s what makes them competent as hackers. With the evolution of threat actor tactics, comes additional pressure for enterprise security experts to match them with new capabilities, even if it means making some big bets on technology solutions that don’t pan out, don’t scale, or don’t solve the fundamental problem immediately. Failure is a thriving environment for technology innovation.
To achieve a mature security program, data science is key.
When building mature security programs, begin with the end in mind. Cyber resilience and maturity go hand in hand. An enterprise with the ability to recover quickly from security incidents, apply the learnings from those security incidents, and minimize the business impact is as good as it gets. Cyber resilience not only applies to the cybersecurity program, but also the entire enterprise.
There are a few foundational components that security program maturity and enterprise resilience are based on. One of the foundational items, which is not necessarily well understood or acknowledged across the industry, is data science. The first person I’ve hired in the past two leadership roles is a data scientist dedicated to the cybersecurity program. There are hundreds of use cases that can be addressed and automated by using data science fundamental constructs.
There are two ways to leverage data science for cybersecurity maturity. The first way, is to apply data science skill to improve data quality for KPI information. And the second way is the game changer: use behavior models to drive frontline security controls without human intervention in near real time.
There are many examples of attempts to use anomaly detection to discover threat actor activities within an enterprise within cybersecurity. The approach that has the highest probability of success is to actually discover the behaviors of legitimate users, and model them using an algorithm. Next, compare behavioral streaming data to the patterns (the algorithm) resulting in a deviation score. Data aligned with a pattern represents the legitimate user. If the deviation score is too high and surpasses a predetermined threshold, then an automated action (eg: revocation of privilege) is triggered. Building behavioral models for every enterprise user enables an enterprise to confirm identity based on the behavioral patterns whenever necessary. If an enterprise user requests a privilege or entitlement that is high risk, then this can trigger the comparison of digital activity compared to the behavioral pattern with a deviation score and threshold predetermined. The behavioral patterns can use attribute information that is considered relatively benign (Geo Location, type of entitlements used most frequently, time of day, etc.).
If you take attribute information and then cluster it into a group, it creates a pattern. Numerically, we can create a deviation score in which we can establish a threshold, let’s just say arbitrarily a 70 and above. Then, you can assign and automate a specific treatment or action for any behavioral deviation score that is 70 and above. By being able to identify thresholds in the deviation score, you can align it with real actions.
In this scenario, we’re eliminating context. Instead, SOC analysts can step back and look across 1000s of transactions and change the threshold scores. Everything I’ve described are basic fundamental data science principles and practices that are relatively straightforward to do, they don’t require a high degree of difficulty, and limited human intervention.
Go beyond risk frameworks.
When I started my cybersecurity career 20 years ago there was a one-size-fits-all model for industry standard cybersecurity practices. We had a validation process where you’d choose a risk framework, align your IT management controls with the control objectives and the risk framework, then hire a third party to do an attestation on how effective your controls are against that framework. If you lined up well, you received a high maturity rating.
Today, we have NIST CSF, 853, ISO 27 001, and other cyber risk frameworks. These risk frameworks are very helpful, practical, and vital tools for an enterprise. But what’s different today is threat actor activity changes rapidly based on adjustments and the effectiveness of established controls. Many threat actors use compromised credentials in credential stuffing attacks on web sites and then monetize the account takeover. Conventional user IDs and passwords have served the enterprise well for 60 years and are reinforced through risk frameworks, but enterprises interested in cyber resilience need to consider designing new controls using data science that ultimately result in improvements in risk frameworks.
Now, the stakeholders applying an industry standard model – CEO, CFO, CIO, board, auditors, regulators, third party governance teams – have bought into this notion of one-size-fits-all to a specific risk framework. The threat actor is the one stakeholder that hasn’t bought in, uses networks of criminal syndicates to improve capabilities, and they are constantly changing and evolving their techniques.
This does not mean that risk frameworks are obsolete, it’s quite the opposite. They are still foundational, they’re just not enough. Enterprises today need the ability to respond to incidents, learn from that, apply those lessons to improve practices, and do this in a continuous way.
I’ve learned to look at the top cyber risks for the enterprise that I’m part of and drive the investment decisions and allocation of resources based on what those risks are. It is important to recognize that any given enterprise may have a different risk profile and attack surface than others, even if they’re in the same industry. Every organization is different. How they make decisions, the cultural norms and behaviors, data management processes, all factors into the attack surface.
My advice to cybersecurity professionals today is embrace those cyber risk frameworks. They’re excellent, and they are a source of wonderful practices. But, they’re not enough by themselves to stay ahead of today’s adversaries. Innovation in control design – perhaps using data science – is essential to achieve cyber resilience and maturity for large enterprises today.
For additional insights on cybersecurity innovation, listen to episode 028 of Agent of Influence.
Explore more blog posts
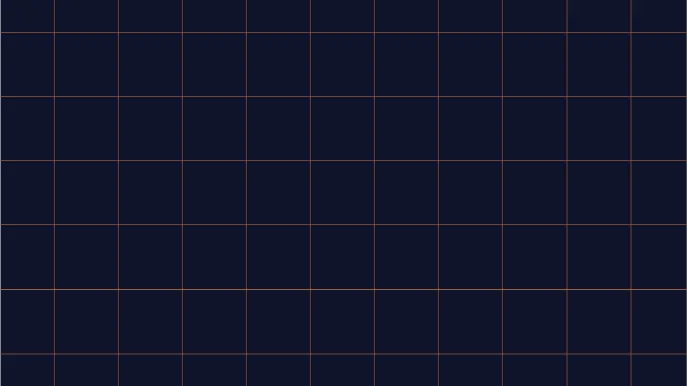
Navigating Cybersecurity Regulations Across Financial Services
Learn about five areas businesses should consider to help navigate cybersecurity regulations, such as the Digital Operations Resiliency Act (DORA).
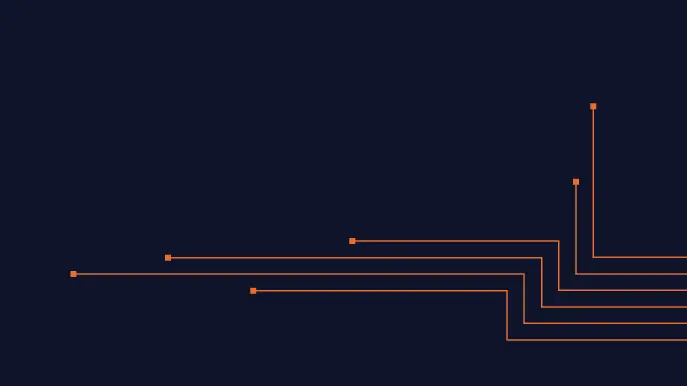
A New Era of Proactive Security Begins: The Evolution of NetSPI
Introducing The NetSPI Platform, the proactive security solution used to discover, prioritize, and remediate the most important security vulnerabilities. Plus, get a first look at NetSPI’s updated brand!
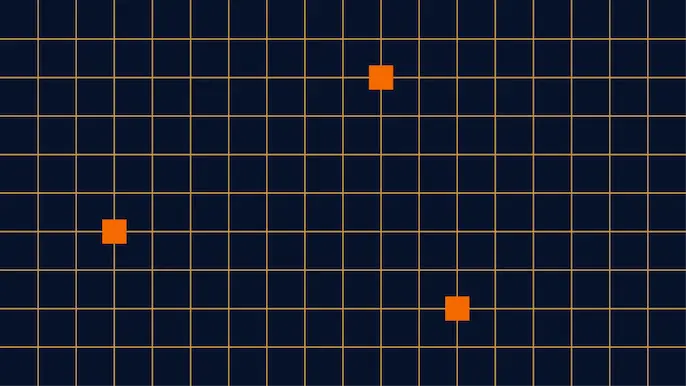
Penetration Testing: What is it?
Learn about 15 types of penetration testing, how pentesting is done, and how to choose a penetration testing company.